Crop Model Calibration
Main Contacts for Initiative
Daniel Wallach (Daniel.Wallach@inrae.fr)
Taru Palosuo (taru.palosuo@luke.fi)
Sabine Seidel (sabine.seidel@uni-bonn.de)
Peter Thorburn (Peter.Thorburn@csiro.au)
Henrike Mielenz (henrike.mielenz@julius-kuehn.de)
Samuel Buis (samuel.buis@inrae.fr)
Brief description of activity
Calibration is arguably the most difficult aspect of applying a crop model to a new problem. The calibration approach is important, because it has a major effect on simulated values. However, there is no consensus as to the best calibration approach, with a wide diversity of practices even for the same model structure. Improving calibration practices is a major pathway for crop model improvement. This calibration activity has three interrelated objectives. 1) Document current calibration practices for crop models. 2) Develop and test protocols for improved calibration practices. 3). Create tools to facilitate implementation of improved calibration practices.
Phase 1 was a web-based survey of calibration practices conducted 2016-2017. In phase 2 (2018-2020), 30 participating modelling groups applied their “usual” calibration practices to two data sets of wheat phenology. The purpose here was to document usual practices and to provide a baseline of prediction accuracy.
In phase 3 (2020-2021), a comprehensive (i.e. covering all steps of the calibration activity), generic (i.e. applicable to all models) protocol for calibration using phenology data was proposed and tested. A major innovation compared to usual methods was the introduction of a test for choosing which parameters to estimate, based on the corrected Akaike Information Criterion (AICc), which protects against over-fitting. It was found that when all groups used the protocol, both the variability between groups and average prediction error were reduced compared to usual calibration.
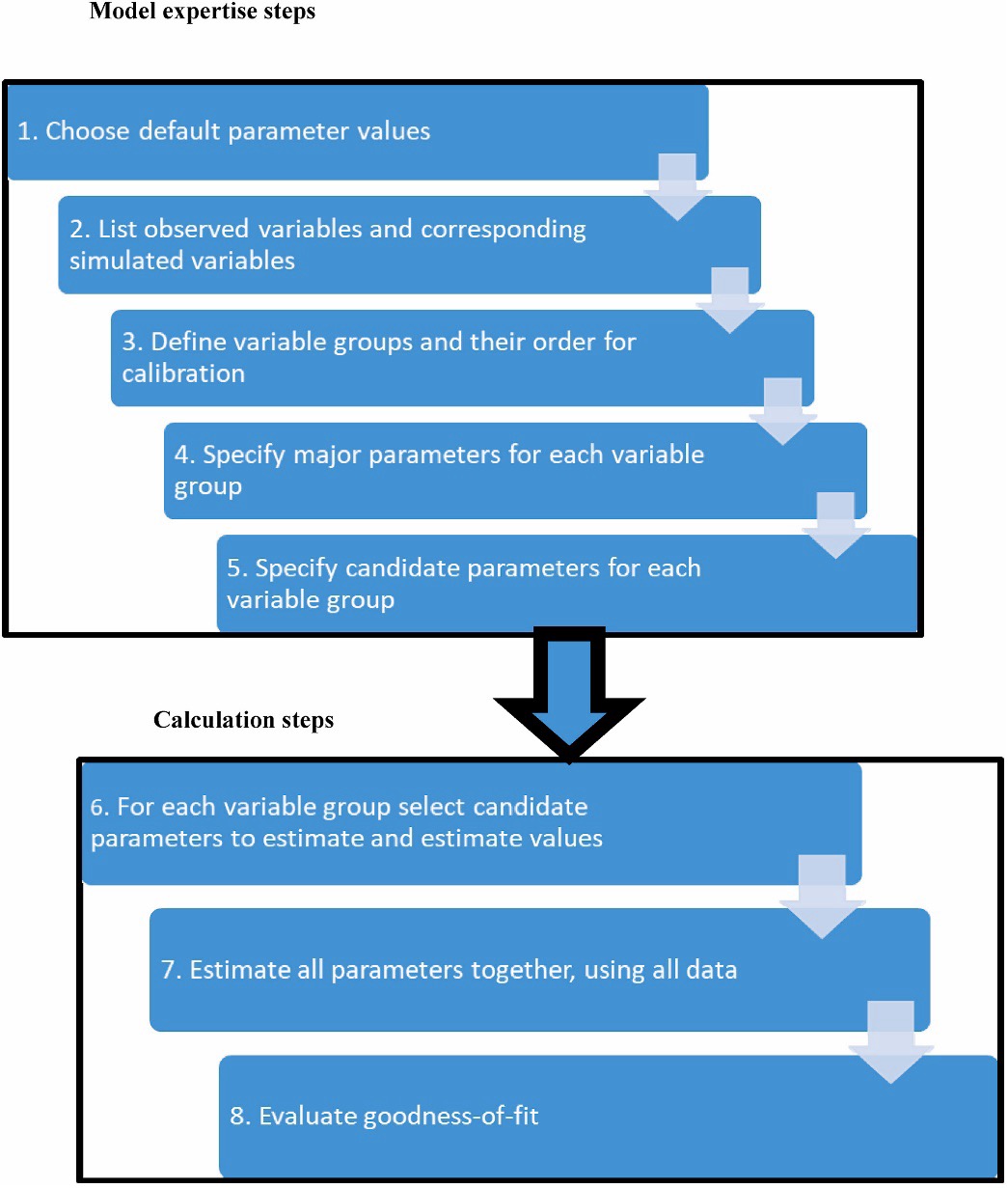
Schema of calibration protocol. The first five steps involve codifying model expertise. Given that information, the calculation steps 6–8 require no farther model-specific inputs. Taken from Wallach et al. (2024) https://doi.org/10.1016/j.envsoft.2024.106147
In phase 4, building on the results for phenology, a protocol applicable to any plant or soil data was proposed. The protocol was tested using artificial data and then tested with field data and a single model. A multi-model study applying the protocol is currently underway. The protocol is directly based on statistical methods of parameter estimation in regression. The choice of parameters is based on forward regression with AICc as the criterion for including a parameter in the list of parameters to estimate. The diversity of variables (e.g. phenology, biomass, yield) is handled using weighted least squares (WLS). An initial approximation to the WLS parameters is obtained by first estimating parameters for one variable group at a time. The protocol includes recommendations for documentation to improve transparency and collaboration. It also includes diagnostic tests to evaluate model-specific decisions about the order of variable groups and the choice of bias-reducing parameters. Software solutions based on the use of the generic packages CroptimizR and CroPlotR have been implemented to apply the calibration protocols to various models in phases 3 and 4 of the project. A new version of the CroptimizR package fully integrating the protocol proposed in phase IV is currently being implemented to facilitate its application to any dataset.
Current Research Focus
The current focus is on testing the phase 4 protocol. It needs to be tested with multiple models and with multiple different data structures.
Recent Noteworthy Finding
- The variability in multi-model simulation results was found to be due more to variability in parameter values than to variability in model structure, in two case studies.
- A comprehensive, generic crop model calibration protocol was proposed .
- The proposed protocol performed quite well for an artificial data example with STICS and with field data, for the DSSAT-NWheat model.
- The application of the protocol requires model expertise, but diagnostics have been proposed to evaluate the model-specific decisions.
- Software tools based on the use of the generic packages CroptimizR and CroPlotR have been implemented to apply the calibration protocols to various models.
Recent Papers/Reports
Wallach, D., Kim, K. S.; Hyun, S., Buis, S. Mielenz, H., Seidel, S.J., Thorburn, P., Alderman, P., Dumont, B., Fallah, M. H., Hoogenboom, G., Justes, E., Kersebaum, K.-C., Launay, M., Leolini, L., Mehmood, M. Z., Moriondo, M., Jing, Q., Qian, B., Schulz, S., Seserman, D.-M., Shelia, V., Weihermüller, L., Palosuo, T. 2025. The Agmip calibration protocol for soil-crop models; Case study and new diagnostic tests. Available at SSRN. http://dx.doi.org/10.2139/ssrn.5110527 · Wallach, D., Kim, K. S.; Hyun, S., Buis, S. Mielenz, H., Seidel, S.J., Thorburn, P., Alderman, P., Dumont, B., Fallah, M. H., Hoogenboom, G., Justes, E., Kersebaum, K.-C., Launay, M., Leolini, L., Mehmood, M. Z., Moriondo, M., Jing, Q., Qian, B., Schulz, S., Seserman, D.-M., Shelia, V., Weihermüller, L., Palosuo, T. 2025. The Agmip calibration protocol for soil-crop models; Case study and new diagnostic tests. Available at SSRN. http://dx.doi.org/10.2139/ssrn.5110527
Wallach, D., Palosuo, T., Mielenz, H., Buis, S., Thorburn, P., Asseng, S., Dumont, B., Ferrise, R., Gayler, S., Ghahramani, A., Harrison, M. T., Hochman, Z., Hoogenboom, G., Mingxia, H., Jing, Q., Justes, E., Kersebaum, K.-C., Launay, M., Lewan, E., Liu, K., Luo, Q., Mequanint, F., Nendel, C., Padovan, G., Olesen, J. E., Pullens, J. W. M., Qian, B., Seserman, D.-M., Shelia, V., Souissi, A., Specka, X., Wang, J., Weber, T.K.D., Weihermüller, L., Seidel, S.J., 2024, Why is there so much variability in crop multi-model studies? Available at Biorxiv. https://doi.org/10.1101/2023.02.03.526931
Wallach, D., Buis, S., Seserman, D.-M., Palosuo, T., Thorburn, P. J., Mielenz, H., Justes, E., Kersebaum, K.-C., Dumont, B., Launay, M., and S. J. Seidel, 2024: A calibration protocol for soil-crop models. Environmental Modelling & Software. 180, 106147. https://doi.org/10.1016/j.envsoft.2024.106147
Wallach, D., Palosuo, T., Thorburn, P., Mielenz, H., Buis, S., Hochman, Z., Gourdain, E., Andrianasolo, F., Dumont, B., Ferrise, R., Gaiser, T., Garcia, C., Gayler, S., Harrison, M., Hiremath, S., Horan, H., Hoogenboom, G., Jansson, P.-E., Jing, Q., Justes, E., Kersebaum, K.-C., Launay, M., Lewan, E., Liu, K., Mequanint, F., Moriondo, M., Nendel, C., Padovan, G., Qian, B., Schütze, N., Seserman, D.-M., Shelia, V., Souissi, A., Specka, X., Srivastava, A.K., Trombi, G., Weber, T.K.D., Weihermüller, L., W¨ohling, T., Seidel, S.J., 2023. Proposal and extensive test of a calibration protocol for crop phenology models. Agron. Sustain. Dev. 43, 46, https://doi.org/10.1007/s13593-023-00900-0
Wallach, D., Palosuo, T., Thorburn, P., Gourdain, E., Asseng, S., Basso, B., Buis, S., Crout, N., Dibari, C., Dumont, B., Ferrise, R., Gaiser, T., Garcia, C., Gayler, S., Ghahramani, A., Hochman, Z., Hoek, S., Hoogenboom, G., Horan, H., Huang, M., Jabloun, M., Jing, Q., Justes, E., Kersebaum, K.C., Klosterhalfen, A., Launay, M., Luo, Q., Maestrini, B., Mielenz, H., Moriondo, M., Nariman Zadeh, H., Olesen, J.E., Poyda, A., Priesack, E., Pullens, J.W.M., Qian, B., Schütze, N., Shelia, V., Souissi, A., Specka, X., Srivastava, A.K., Stella, T., Streck, T., Trombi, G., Wallor, E., Wang, J., Weber, T.K.D., Weihermüller, L., de Wit, A., Wöhling, T., Xiao, L., Zhao, C., Zhu, Y., Seidel, S.J., 2021a. How well do crop modeling groups predict wheat phenology, given calibration data from the target population? Eur. J. Agron. 124, 126195. https://doi.org/https://doi.org/10.1016/j.eja.2020.126195
Wallach, D., Palosuo, T., Thorburn, P., Hochman, Z., Andrianasolo, F., Asseng, S., Basso, B., Buis, S., Crout, N., Dumont, B., Ferrise, R., Gaiser, T., Gayler, S., Hiremath, S., Hoek, S., Horan, H., Hoogenboom, G., Huang, M., Jabloun, M., Jansson, P.-E., Jing, Q., Justes, E., Kersebaum, K.C., Launay, M., Lewan, E., Luo, Q., Maestrini, B., Moriondo, M., Padovan, G., Olesen, J.E., Poyda, A., Priesack, E., Pullens, J.W.M., Qian, B., Schütze, N., Shelia, V., Souissi, A., Specka, X., Srivastava, A.K., Stella, T., Streck, T., Trombi, G., Wallor, E., Wang, J., Weber, T.K.D., Weihermüller, L., Wit, A. de, Wöhling, T., Xiao, L., Zhao, C., Zhu, Y., Seidel, S.J., 2021b. Multi-model evaluation of phenology prediction for wheat in Australia. Agric. For. Meteorol. 298–299, 108289 https://doi.org/10.1101/2020.06.06.133504
Wallach, D., Palosuo, T., Thorburn, P., Hochman, Z., Gourdain, E., Andrianasolo, F., Asseng, S., Basso, B., Buis, S., Crout, N., Dibari, C., Dumont, B., Ferrise, R., Gaiser, T., Garcia, C., Gayler, S., Ghahramani, A., Hiremath, S., Hoek, S., Horan, H., Hoogenboom, G., Huang, M., Jabloun, M., Jansson, P.-E., Jing, Q., Justes, E., Kersebaum, K.C., Klosterhalfen, A., Launay, M., Lewan, E., Luo, Q., Maestrini, B., Mielenz, H., Moriondo, M., Zadeh, H.N., Padovan, G., Olesen, J.E., Poyda, A., Priesack, E., Pullens, J.W.M., Qian, B., Schütze, N., Shelia, V., Souissi, A., Specka, X., Srivastava, A.K., Stella, T., Streck, T., Trombi, G., Wallor, E., Wang, J., Weber, T.K.D., Weihermüller, L., Wit, A. de, Wöhling, T., Xiao, L., Zhao, C., Zhu, Y., Seidel, S.J., 2021c. The chaos in calibrating crop models: lessons learned from a multi-model calibration exercise. Environ. Model. Software 145, 105206. https://doi.org/10.1016/J.ENVSOFT.2021.105206
Seidel, S.J., T. Palosuo, P. Thorburn, and D. Wallach, 2018: Towards improved calibration of crop models – Where are we now and where should we go? European Journal of Agronomy, Volume 94, March 2018, Pages 25-35, https://doi.org/10.1016/j.eja.2018.01.006