AgMIP NextGen Research Pillar Featured in Agricultural Systems Special Issue
Aug 17, 2017
By: Greg Reppucci
In July 2017, Agricultural Systems released a special issue on the Next Generation Data, Models and Knowledge Products. Focusing on the Agricultural Model Intercomparison and Improvement Project (AgMIP) Next Generation (NextGen) research pillar, this special issue provides insights in the current state of agricultural system models, the future visions of agricultural systems modeling, and the topics that must be addressed to turn this vision into reality. Thanks to the generous contribution of The Bill and Melinda Gates Foundation, the special issue is available for public access via open agreements and is available for download here.
Agricultural Systems invited AgMIP Principal Investigators John Antle, James Jones, and Cynthia Rosenzweig to guest-edit a special issue on the next generation of agricultural systems data, models and knowledge products. In their introduction, Antle, Jones & Rosenzweig acknowledge that while agricultural systems models have become important in providing valuable information to decision makers, there is potential for these models to improve substantially. Most notably, the recent advancements in data, information, and communications technology have yet to be adopted by agricultural system data, models, and knowledge products.
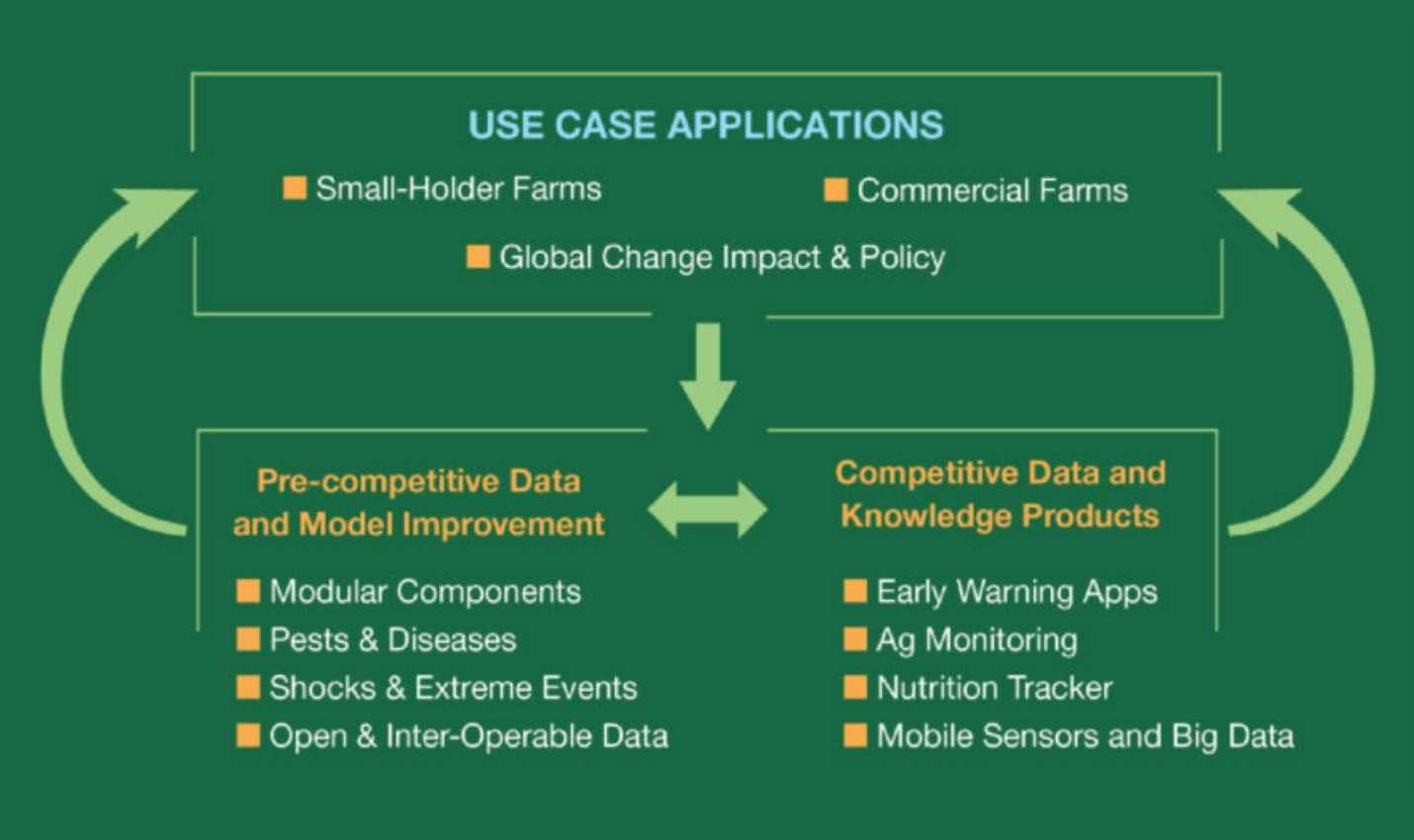
The image above documents the components associated with the NextGen strategy. This strategy will encourage the development of NextGen data, models, and knowledge tools to support decision makers. (From Antle, Jones, and Rosenzweig, 2017, Next generation agricultural system models and knowledge products: Synthesis and strategy)
There is a great opportunity for agriculturally focused information and communications technology (ICT) tools to be created. These tools can especially help in improving the well-being of both smallholder farm households in the developing world, as well as commercial farmers in the industrial world.
New ICT tools should be developed in a manner that allows for a wide audience to use them. To achieve this, Antle, Jones & Rosenzweig stress how research and development must focus on the needs of the user community. This “demand-driven” approach will encourage knowledge products to be more accessible and easier to comprehend. In this way, these new tools will improve informed decision-making.
To assess the gaps between current model outputs and decision-maker needs, the AgMIP research team developed five Use Cases as a method to consider a spectrum of potential model end-users. These Use Cases, developed during a stakeholder workshop sponsored by the Gates Foundation, highlight five potential model output users and analyze current and future model development through the user perspective. In this way, specific information and decision contexts of diverse users are anticipated as part of the analysis. “This workshop was an important part of the AgMIP NextGen project,” explains Antle. “It confirmed the value of the use-case approach the NextGen author teams used to evaluate the state of the science and the challenges before us.”
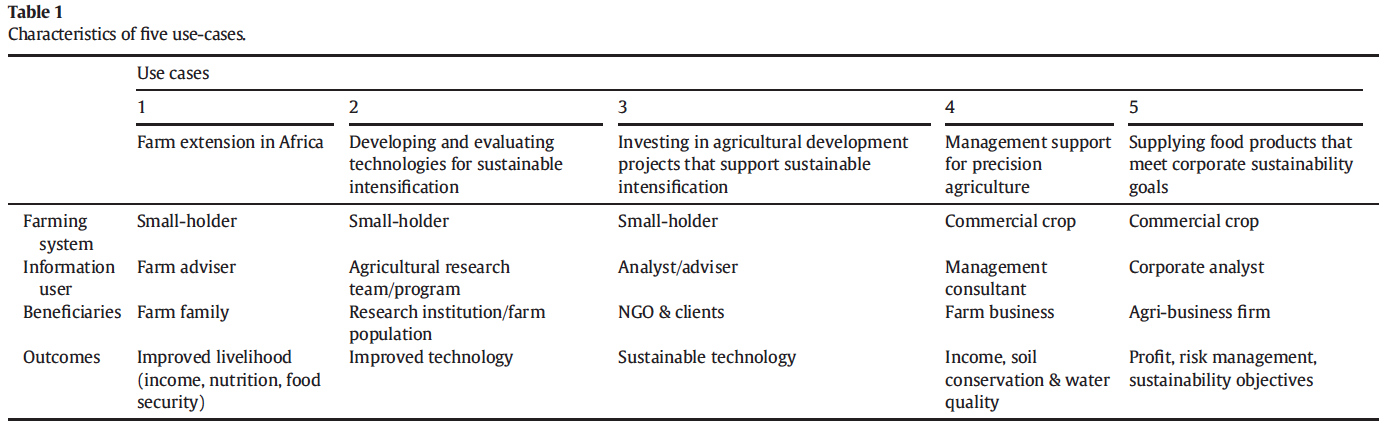
The NextGen Study utilized 5 unique Use Cases to represent the end-user/demand-driven perspective. These Use Cases were developed during a stakeholder workshop hosted by the Gates Foundation. (from Antle, Jones, and Rosenzweig, 2017, Next generation agricultural system data, models and knowledge products: Introduction).
This special issue builds off the foundational work established during the stakeholder workshop. Four papers provide a foundation for the development of the Next Generation of models, while the remaining three address topical issues currently found in agricultural systems modeling.
The first paper, Brief history of agricultural systems modeling by Jones et al., provides a historical overview of biophysically-based models and agriculturally associated economic models. Modeling has experienced periods of stagnant development and relatively quick advancement. These advancements in agricultural systems modeling were fastest after certain events caused environmental or economic concern. Technological advances have had major benefits on agricultural system modeling, significantly improving their use. Though data availability has improved, there is still a significant gap in data today and current data limitations hinder model advancements substantially. The strength of models has improved in recent history, and the availability of open, harmonized data will continue to improve this robustness.
In Toward a new generation of agricultural system data, models, and knowledge products: State of agricultural systems science, Jones et al. shifts focus to provide an in-depth view of different model systems and most commonly used approaches to modeling. The authors analyze multiple components of biophysical and economic models and show data limitations are prevalent throughout modeling systems. The modeling approaches are also analyzed from the perspective of the 5 Use Cases adopted by the foundational research articles of the special issue, enabling the specific consideration of critical gaps between current model outputs and the needed outputs from an array of stakeholders who could benefit.
Antle et al., in Towards a new generation of agricultural system data, models and knowledge products: Design and improvement, consider how shifts in perspectives may improve the extent to which rigorous scientific research considers end-user needs. Model development could begin with a consideration of end-user needs, before working backwards to determining what data and models are required to deliver these needs. This restructuring of model development will necessitate a new institutional and incentive-based arrangement that will involve a collaborative “pre-competitive” space for model development and “competitive space” for knowledge product development.
In Towards a new generation of agricultural system data, models, and knowledge products: Information and communication technology, Janssen et al. address the capabilities for information and communications technology (ICT) tools to benefit agricultural systems models and knowledge products. In particular, the authors provide a framework from which models can be created in a faster, cheaper, and more effective manner. An open access, web-based approach will not only provide more individuals with access to models, but also will help supply scientists with significantly more data, and will help in reducing current data gaps and limitations. For this to happen, models must become simpler in their coding, their user-face must be easier to understand to allow for more individuals to engage with the models, and a set of standards must be established to ensure the products continue to develop in a universal way.
Following the foundational research articles for the next generation of models, the special issue turns to address three topical research articles of great importance to the overall advancement of agricultural systems modeling.
In Modelling the Impacts of Pests and Diseases , Donatelli et al. note that improvement of model ability to simulate the effects of pests and diseases on crops can vastly improve agricultural systems modeling all together. A 5-step roadmap to improving pest and disease modeling outputs is laid out. The roadmap highlights the need for improved linkages between pest/disease models and crop models to effectively achieve integration of these models. The coupling point – the point at which pest models and crop models interact with one another (i.e., where data is transferred) – is critical to this integration, and the impact of these models going forward.
Capalbo, Antle, and Seavert, in Next Generation data systems and knowledge products to support agricultural producers and science-based policy decision making, provide an example of a forward-thinking approach to linking farm-level decision models with larger landscape-scale policy analysis. Data access is important for this linkage to succeed, and the authors suggest developing “cloud-based” software as a method to achieving the data gap. Ideally, robust datasets will encourage farm-based data and tools to feed information and indicators into these landscape-scale tools. Two analytical tools – AgBiz Logic and TOA-MD – can demonstrate the farm-based, landscape-scale relationship.
In Next generation crop models: A modular approach to model early vegetative and reproductive development of the common bean (Phaseolus vulgaris L) authors Hwang et al. discuss improving model parameterization and methods to integrating genetic information into crop models. Incorporating genetic information into crop models could be very valuable, as it may ultimately provide insight in determining the most appropriate genotypes (genetic variety of a plant) for certain environments. Being able to model crops while incorporating phenotype information and its interaction with environmental factors (such as amount of day light and temperature), would greatly improve the amount of available information. The authors demonstrate this by providing an example approach simulating changes in the main stem of the common bean.
Antle, Jones, and Rosenzweig conclude this special issue by offering their editorial comments in Next generation agricultural system models and knowledge products: Synthesis and strategy. The guest editors stress how important it is to close the current data availability gap in agricultural systems modeling. The lack of access to data will continue to hinder the development and effectiveness of these models, but extensive collaboration amongst communities, they suggest, could resolve this issue rather rapidly. In improving the relevance of models for end-users – by increasing transparency, linking to context-specific data, and developing user-friendly applications –significant improvements can be possible for the new generation. Providing additional outcomes beyond crop yields, such as nutrition, environmental outcomes, and health indicators, will vastly improve systems model use.
“Rapidly advancing data and IT capabilities present great opportunities to create the next generation of agricultural systems model,” Says Antle. “These models can be key tools to advance both science and informed decision making.”
Achieving the next generation of agricultural systems models and knowledge products requires a forward-thinking strategy. This strategy requires approaching model development through a demand-driven perspective. Prioritizing the needs of end users will ultimately cause key data and output gaps to fill, will drive the investments necessary for model development, and will ultimately provide opportunity for collaboration between the “pre-competitive” data and model improvement/ public good space, and the “competitive” data and knowledge products / private good space. While the “competitive” space primarily consists of private goods, the “pre-competitive” space occupied by data and inputs should not be overlooked. Linking these two spaces will allow for a greater amount of data and inputs to be available in the improvement and development of products. AgMIP’s commitment to focusing on both the science and application of data and models will help establish this link between spaces. It will encourage overlap in the next generation of model development.